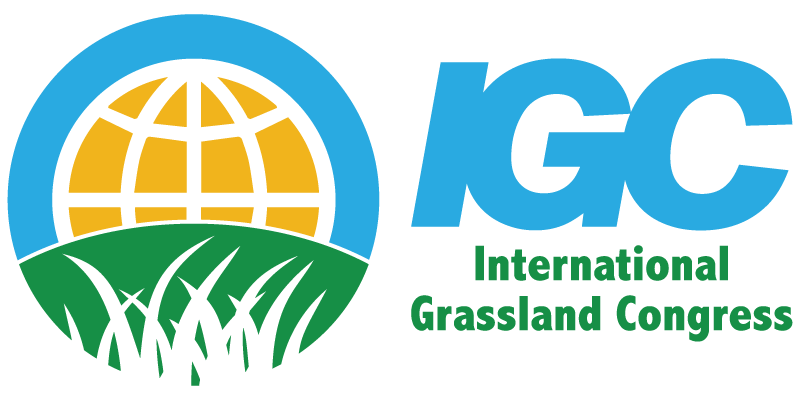
Theme 1-1: Rangeland/Grassland Ecology--Oral Sessions
Description
Dry season plant biomass is critical for livestock production and hence livelihoods in rangeland communities. We have developed a cloud-based application that employs remote sensing data to provide weekly spatially explicit information on plant vegetation cover in West Africa during the dry season (typically October-June). In this paper, we discuss the data analysis steps and results that drive the application. Linear spectral mixture analysis is used to derive endmember samples of basic landcover primitives (active/green vegetation, non-active vegetation, and bare soil) from very high-resolution imagery that spans the spatiotemporal spectrum from wet/peak-green to dry/dormant conditions in Senegal. These samples are used to train and evaluate ensemble tree models for predicting proportional cover of the same land cover primitives at 500m scale, using MODIS derived NDVI, shortwave infra-red bands 3 and 2 (SWIR3 and SWIR2), and total 15-day antecedent precipitation as predictors. Our trained models can predict the fractional cover of green vegetation, non-green vegetation and bare soil across space and time with cross-validation root-mean square errors of 12%, 15% and 9% respectively. With a weekly cadence and low latency (~2-3 weeks), the tool can also provide timely information to support local decision making in the management of critical rangeland resources.
Citation
Anchang, J. Y.; Ross, C. W.; Ji, W.; Yu, Q.; Lind, B.; Prihodko, L.; and Hanan, N. P., "Short-Term Dry Season Forage Monitoring in Rangelands and Savannas of West Africa" (2021). IGC Proceedings (1989-2023). 41.
https://uknowledge.uky.edu/igc/24/1/41
Short-Term Dry Season Forage Monitoring in Rangelands and Savannas of West Africa
Dry season plant biomass is critical for livestock production and hence livelihoods in rangeland communities. We have developed a cloud-based application that employs remote sensing data to provide weekly spatially explicit information on plant vegetation cover in West Africa during the dry season (typically October-June). In this paper, we discuss the data analysis steps and results that drive the application. Linear spectral mixture analysis is used to derive endmember samples of basic landcover primitives (active/green vegetation, non-active vegetation, and bare soil) from very high-resolution imagery that spans the spatiotemporal spectrum from wet/peak-green to dry/dormant conditions in Senegal. These samples are used to train and evaluate ensemble tree models for predicting proportional cover of the same land cover primitives at 500m scale, using MODIS derived NDVI, shortwave infra-red bands 3 and 2 (SWIR3 and SWIR2), and total 15-day antecedent precipitation as predictors. Our trained models can predict the fractional cover of green vegetation, non-green vegetation and bare soil across space and time with cross-validation root-mean square errors of 12%, 15% and 9% respectively. With a weekly cadence and low latency (~2-3 weeks), the tool can also provide timely information to support local decision making in the management of critical rangeland resources.