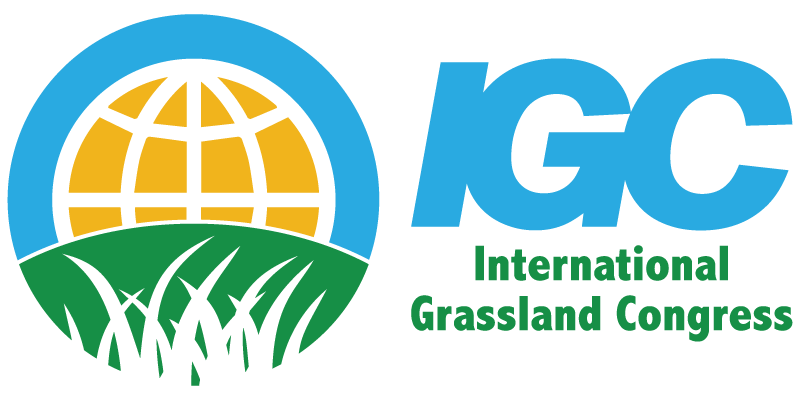
Track 2-3-2: Impact of Grass and Legumes on Livestock Production, Soil Conservation and the Nitrogen Cycle in Grassland and Forage Ecosystems
Description
Stable isotope measurements have been increasingly used as a method to obtain information on relationships between plants and their environment (Dawson et al., 2002). Stable isotopes are seen as a powerful tool for advancing our knowledge on stock cycling and, nitrogen and carbon isotopic compositions have provided key insights into biogeochemical interactions between plants, soils and the atmosphere (Robinson, 2001). For the stable isotope measurements, the δ13C isotopic signature has been used successfully to disentangle physiological, ecological and biogeochemical processes and, δ15N studies have significantly improved our knowledge on nitrogen cycling pathways and nitrogen acquisition by plants (Vallano and Sparks, 2008).
For the stable isotope measurements, traditional laboratory methods using isotope analysis are accurate and reliable, but usually time-consuming and expensive. Near-infrared spectroscopy (NIRS) analysis provides rapid, accurate and less expensive estimation. NIRS have been made to estimate herbage parameters using statistical methods such as multiple linear regression and partial least square regression (PLSR). PLSR uses all available wavebands in multivariate calibration for quantitative analysis of the spectral data. However, previous studies indicated that PLSR with waveband selection might improve their predictive accuracy in multivariate calibration at laboratory (Leardi, 2000) and the selection of appropriate wavelengths can refine the predictive accuracy of the PLS model by optimizing important spectral wavebands both in laboratory NIRS (Jiang et al., 2002). To optimize important spectral wavebands by wavelength selection, genetic algorithms (GA) is widely used, because GA has the ability to simulate the natural evolution of an individual and GA is well suited for solving variable subset selection problems (Ding et al., 1998).
Barley and pea mixture is one of the most important forage species for livestock farming in Korea. To investigate nitrogen fixation and transfer in barley and pea mixture, stable isotope measurements was widely used. However, there was no research to estimate stable isotope in barley and pea mixture using NIRS in Korea. The aim of this study was to investigate performance of NIRS with PLSR using genetic algorithms based wavelength selection (GA-PLSR) and compare with PLSR without wavelength selection (FS-PLSR) for the estimation of δ15N and δ13C in barley and pea mixture.
Citation
Lee, Hyo-Won; Lee, Hyo-Jin; Ko, Han-Jong; and Yoon, Chang, "Estimating δ15N and δ13C in Barley and Pea Mixtures Using Near-Infrared Spectroscopy with Genetic Algorithm Based Partial Least Squares Regression" (2020). IGC Proceedings (1993-2023). 6.
https://uknowledge.uky.edu/igc/23/2-3-2/6
Included in
Estimating δ15N and δ13C in Barley and Pea Mixtures Using Near-Infrared Spectroscopy with Genetic Algorithm Based Partial Least Squares Regression
Stable isotope measurements have been increasingly used as a method to obtain information on relationships between plants and their environment (Dawson et al., 2002). Stable isotopes are seen as a powerful tool for advancing our knowledge on stock cycling and, nitrogen and carbon isotopic compositions have provided key insights into biogeochemical interactions between plants, soils and the atmosphere (Robinson, 2001). For the stable isotope measurements, the δ13C isotopic signature has been used successfully to disentangle physiological, ecological and biogeochemical processes and, δ15N studies have significantly improved our knowledge on nitrogen cycling pathways and nitrogen acquisition by plants (Vallano and Sparks, 2008).
For the stable isotope measurements, traditional laboratory methods using isotope analysis are accurate and reliable, but usually time-consuming and expensive. Near-infrared spectroscopy (NIRS) analysis provides rapid, accurate and less expensive estimation. NIRS have been made to estimate herbage parameters using statistical methods such as multiple linear regression and partial least square regression (PLSR). PLSR uses all available wavebands in multivariate calibration for quantitative analysis of the spectral data. However, previous studies indicated that PLSR with waveband selection might improve their predictive accuracy in multivariate calibration at laboratory (Leardi, 2000) and the selection of appropriate wavelengths can refine the predictive accuracy of the PLS model by optimizing important spectral wavebands both in laboratory NIRS (Jiang et al., 2002). To optimize important spectral wavebands by wavelength selection, genetic algorithms (GA) is widely used, because GA has the ability to simulate the natural evolution of an individual and GA is well suited for solving variable subset selection problems (Ding et al., 1998).
Barley and pea mixture is one of the most important forage species for livestock farming in Korea. To investigate nitrogen fixation and transfer in barley and pea mixture, stable isotope measurements was widely used. However, there was no research to estimate stable isotope in barley and pea mixture using NIRS in Korea. The aim of this study was to investigate performance of NIRS with PLSR using genetic algorithms based wavelength selection (GA-PLSR) and compare with PLSR without wavelength selection (FS-PLSR) for the estimation of δ15N and δ13C in barley and pea mixture.