Abstract
It is of practical significance to understand the specific impact of weather events on the operating condition of the surface transportation system so that proactive and reactive strategies can be quickly implemented by transportation agencies to minimize the negativity resulted from adverse weather events. Many studies have been conducted on quantifying such effects yet suffer from limitations such as subjectively defining a time window under uncongested conditions and not being able to account for the severe impact from weather events which result in travel time unreliability. To overcome those shortcomings in existing literature, an integrated data mining framework based on decision tree and quantile regression techniques is developed in this study. The results demonstrate that the approach is effective in characterizing time periods with different traffic characteristics and quantifying the impact of rain and snow events on both congestion and reliability aspects of the transportation system. It is observed that snow events impose more significant impact on travel times than that from rain events. In addition, the impact from weather events is even more severe on travel time reliability than average delay. The impact magnitude is directly related to the level of recurrent congestion under study. Other insights with regard to the capability of quantile regression and future improvement on the methodological design are also offered.
Document Type
Article
Publication Date
3-3-2019
Digital Object Identifier (DOI)
https://doi.org/10.1155/2019/8203081
Repository Citation
Zhang, Xu and Chen, Mei, "Quantifying the Impact of Weather Events on Travel Time and Reliability" (2019). Kentucky Transportation Center Faculty and Researcher Publications. 14.
https://uknowledge.uky.edu/ktc_facpub/14
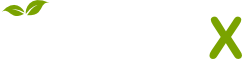
- Citations
- Citation Indexes: 33
- Policy Citations: 1
- Usage
- Downloads: 70
- Abstract Views: 45
- Captures
- Readers: 54
Notes/Citation Information
Published in Journal of Advanced Transportation, v. 2019, article ID 8203081, p. 1-9.
Copyright © 2019 Xu Zhang and Mei Chen.
This is an open access article distributed under the Creative Commons Attribution License, which permits unrestricted use, distribution, and reproduction in any medium, provided the original work is properly cited.