Abstract
The goal of human-robot motion retargeting is to let a robot follow the movements performed by a human subject. Typically in previous approaches, the human poses are precomputed from a human pose tracking system, after which the explicit joint mapping strategies are specified to apply the estimated poses to a target robot. However, there is not any generic mapping strategy that we can use to map the human joint to robots with different kinds of configurations. In this paper, we present a novel motion retargeting approach that combines the human pose estimation and the motion retargeting procedure in a unified generative framework without relying on any explicit mapping. First, a 3D parametric human-robot (HUMROB) model is proposed which has the specific joint and stability configurations as the target robot while its shape conforms the source human subject. The robot configurations, including its skeleton proportions, joint limitations, and DoFs are enforced in the HUMROB model and get preserved during the tracking procedure. Using a single RGBD camera to monitor human pose, we use the raw RGB and depth sequence as input. The HUMROB model is deformed to fit the input point cloud, from which the joint angle of the model is calculated and applied to the target robots for retargeting. In this way, instead of fitted individually for each joint, we will get the joint angle of the robot fitted globally so that the surface of the deformed model is as consistent as possible to the input point cloud. In the end, no explicit or pre-defined joint mapping strategies are needed. To demonstrate its effectiveness for human-robot motion retargeting, the approach is tested under both simulations and on real robots which have a quite different skeleton configurations and joint degree of freedoms (DoFs) as compared with the source human subjects.
Document Type
Article
Publication Date
4-18-2019
Digital Object Identifier (DOI)
https://doi.org/10.1109/ACCESS.2019.2911883
Funding Information
This work was supported in part by the USDA under Grant 2018-67021-27416, in part by the NSF under Grant IIP-1543172, in part by the NSFC under Grant 51475373 and Grant 61603302, and in part by the Key Industrial Innovation Chain of Shaanxi Province Industrial Area under Grant 2016KTZDGY06-01.
Repository Citation
Wang, Sen; Zuo, Xinxin; Wang, Runxiao; and Yang, Ruigang, "A Generative Human-Robot Motion Retargeting Approach Using a Single RGBD Sensor" (2019). Computer Science Faculty Publications. 33.
https://uknowledge.uky.edu/cs_facpub/33
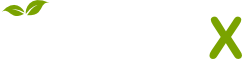
- Citations
- Citation Indexes: 7
- Patent Family Citations: 1
- Usage
- Downloads: 86
- Abstract Views: 18
- Captures
- Readers: 27
Notes/Citation Information
Published in IEEE Access, v. 7, p. 51499-51512.
© 2019 IEEE
The copyright holder has granted the permission for posting the article here.